Summary
Maintaining a single source of truth for your data is the biggest challenge in data engineering. Different roles and tasks in the business need their own ways to access and analyze the data in the organization. In order to enable this use case, while maintaining a single point of access, the semantic layer has evolved as a technological solution to the problem. In this episode Artyom Keydunov, creator of Cube, discusses the evolution and applications of the semantic layer as a component of your data platform, and how Cube provides speed and cost optimization for your data consumers.
Announcements
- Hello and welcome to the Data Engineering Podcast, the show about modern data management
- This episode is brought to you by Datafold – a testing automation platform for data engineers that prevents data quality issues from entering every part of your data workflow, from migration to dbt deployment. Datafold has recently launched data replication testing, providing ongoing validation for source-to-target replication. Leverage Datafold's fast cross-database data diffing and Monitoring to test your replication pipelines automatically and continuously. Validate consistency between source and target at any scale, and receive alerts about any discrepancies. Learn more about Datafold by visiting dataengineeringpodcast.com/datafold.
- Dagster offers a new approach to building and running data platforms and data pipelines. It is an open-source, cloud-native orchestrator for the whole development lifecycle, with integrated lineage and observability, a declarative programming model, and best-in-class testability. Your team can get up and running in minutes thanks to Dagster Cloud, an enterprise-class hosted solution that offers serverless and hybrid deployments, enhanced security, and on-demand ephemeral test deployments. Go to dataengineeringpodcast.com/dagster today to get started. Your first 30 days are free!
- Data lakes are notoriously complex. For data engineers who battle to build and scale high quality data workflows on the data lake, Starburst powers petabyte-scale SQL analytics fast, at a fraction of the cost of traditional methods, so that you can meet all your data needs ranging from AI to data applications to complete analytics. Trusted by teams of all sizes, including Comcast and Doordash, Starburst is a data lake analytics platform that delivers the adaptability and flexibility a lakehouse ecosystem promises. And Starburst does all of this on an open architecture with first-class support for Apache Iceberg, Delta Lake and Hudi, so you always maintain ownership of your data. Want to see Starburst in action? Go to dataengineeringpodcast.com/starburst and get $500 in credits to try Starburst Galaxy today, the easiest and fastest way to get started using Trino.
- Your host is Tobias Macey and today I'm interviewing Artyom Keydunov about the role of the semantic layer in your data platform
Interview
- Introduction
- How did you get involved in the area of data management?
- Can you start by outlining the technical elements of what it means to have a "semantic layer"?
- In the past couple of years there was a rapid hype cycle around the "metrics layer" and "headless BI", which has largely faded. Can you give your assessment of the current state of the industry around the adoption/implementation of these concepts?
- What are the benefits of having a discrete service that offers the business metrics/semantic mappings as opposed to implementing those concepts as part of a more general system? (e.g. dbt, BI, warehouse marts, etc.)
- At what point does it become necessary/beneficial for a team to adopt such a service?
- What are the challenges involved in retrofitting a semantic layer into a production data system?
- evolution of requirements/usage patterns
- technical complexities/performance and cost optimization
- What are the most interesting, innovative, or unexpected ways that you have seen Cube used?
- What are the most interesting, unexpected, or challenging lessons that you have learned while working on Cube?
- When is Cube/a semantic layer the wrong choice?
- What do you have planned for the future of Cube?
Contact Info
Parting Question
- From your perspective, what is the biggest gap in the tooling or technology for data management today?
Closing Announcements
- Thank you for listening! Don't forget to check out our other shows. Podcast.__init__ covers the Python language, its community, and the innovative ways it is being used. The Machine Learning Podcast helps you go from idea to production with machine learning.
- Visit the site to subscribe to the show, sign up for the mailing list, and read the show notes.
- If you've learned something or tried out a project from the show then tell us about it! Email hosts@dataengineeringpodcast.com) with your story.
Links
- Cube
- Semantic Layer
- Business Objects
- Tableau
- Looker
- Mode
- Thoughtspot
- LightDash
- Embedded Analytics
- Dimensional Modeling
- Clickhouse
- Druid
- BigQuery
- Starburst
- Pinot
- Snowflake
- Arrow Datafusion
- Metabase
- Superset
- Alation
- Collibra
- Atlan
The intro and outro music is from The Hug by The Freak Fandango Orchestra / CC BY-SA
Sponsored By:
- Starburst: 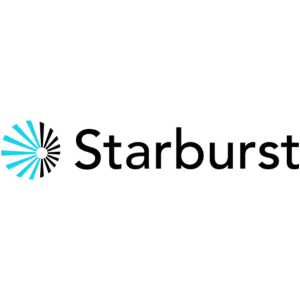 This episode is brought to you by Starburst - a data lake analytics platform for data engineers who are battling to build and scale high quality data pipelines on the data lake. Powered by Trino, Starburst runs petabyte-scale SQL analytics fast at a fraction of the cost of traditional methods, helping you meet all your data needs ranging from AI/ML workloads to data applications to complete analytics. Trusted by the teams at Comcast and Doordash, Starburst delivers the adaptability and flexibility a lakehouse ecosystem promises, while providing a single point of access for your data and all your data governance allowing you to discover, transform, govern, and secure all in one place. Starburst does all of this on an open architecture with first-class support for Apache Iceberg, Delta Lake and Hudi, so you always maintain ownership of your data. Want to see Starburst in action? Try Starburst Galaxy today, the easiest and fastest way to get started using Trino, and get $500 of credits free. [dataengineeringpodcast.com/starburst](https://www.dataengineeringpodcast.com/starburst)
- Datafold: 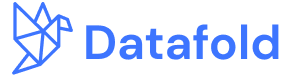 This episode is brought to you by Datafold – a testing automation platform for data engineers that prevents data quality issues from entering every part of your data workflow, from migration to dbt deployment. Datafold has recently launched data replication testing, providing ongoing validation for source-to-target replication. Leverage Datafold's fast cross-database data diffing and Monitoring to test your replication pipelines automatically and continuously. Validate consistency between source and target at any scale, and receive alerts about any discrepancies. Learn more about Datafold by visiting https://get.datafold.com/replication-de-podcast.
- Dagster: 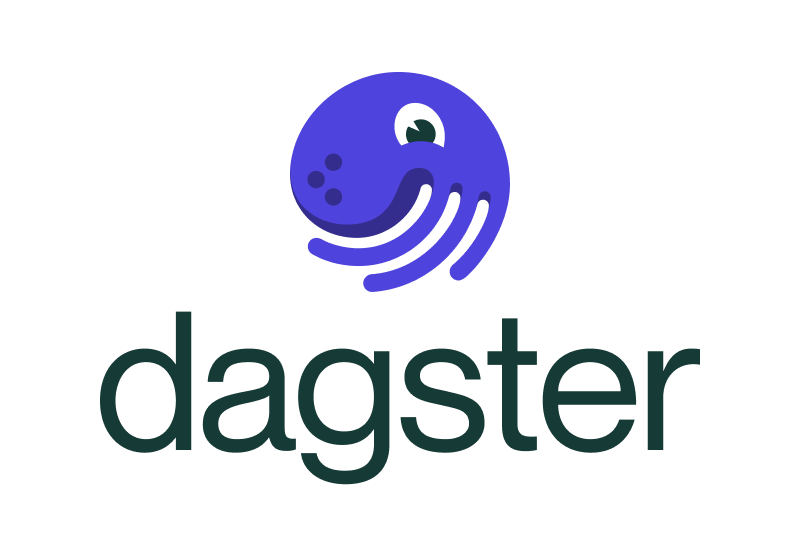 Data teams are tasked with helping organizations deliver on the premise of data, and with ML and AI maturing rapidly, expectations have never been this high. However data engineers are challenged by both technical complexity and organizational complexity, with heterogeneous technologies to adopt, multiple data disciplines converging, legacy systems to support, and costs to manage. Dagster is an open-source orchestration solution that helps data teams reign in this complexity and build data platforms that provide unparalleled observability, and testability, all while fostering collaboration across the enterprise. With enterprise-grade hosting on Dagster Cloud, you gain even more capabilities, adding cost management, security, and CI support to further boost your teams' productivity. Go to [dagster.io](https://dagster.io/lp/dagster-cloud-trial?source=data-eng-podcast) today to get your first 30 days free!